AI Governance Framework: Key Principles & Best Practices

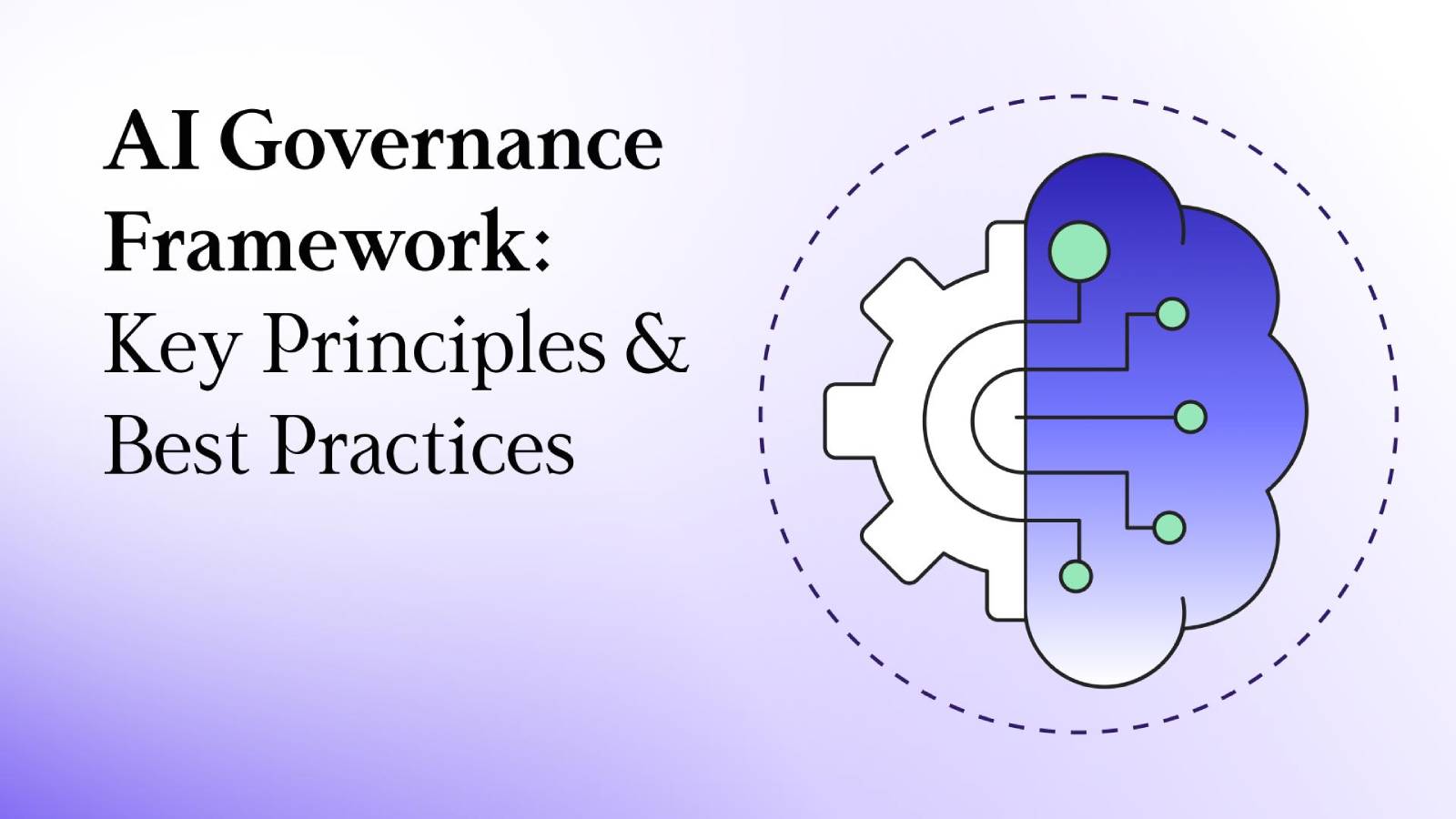
What is AI Governance?
AI governance is the set of policies, processes, and controls that organizations use to ensure the ethical, compliant, and responsible use of AI technologies. It establishes how AI systems are developed, deployed, and monitored to reduce risks such as bias, security threats, and regulatory violations. A strong AI governance framework also ensures that AI decisions are transparent and explainable, staying aligned with organizational values and legal requirements.
AI Governance Examples
Effective AI governance requires practical, real-world applications that demonstrate responsible AI usage across industries. The following examples show how organizations implement governance strategies to manage ethical, compliance, and operational risks:
- AI Model Transparency in Financial Services: A fintech company uses an AI-driven credit scoring system. To meet ethical standards and avoid discrimination, the company establishes explainability protocols, requiring every automated decision to be accompanied by a clear, human-readable explanation. This methodology ensures compliance with the AI Act and builds consumer trust.
- AI Risk Management in Healthcare: A healthcare provider deploying diagnostic AI technologies implements a robust AI governance framework to regularly assess model accuracy and bias. The organization maintains an AI risk register and performs quarterly compliance assessments to meet responsible AI guidelines and regulatory requirements like HIPAA and the upcoming EU AI Act.
- Shadow AI Detection in Enterprise Environments: A global enterprise discovers that employees are using unsanctioned generative AI tools to process sensitive corporate data. By implementing an AI asset discovery solution, the company gains visibility over all deployed AI systems and enforces usage policies, reducing security and compliance risks tied to uncontrolled use of AI.
What is an AI Governance Framework?
An AI governance framework is a structured approach that organizations use to manage the risks, ethics, and compliance requirements associated with AI technologies. This framework defines clear guidelines for the development, deployment, monitoring, and evaluation of AI systems. It helps ensure that AI models produce fair and transparent outcomes while meeting legal and organizational standards.
AI Governance Framework vs. Data Governance Framework
While both frameworks aim to improve decision-making and manage risks, they focus on different domains within the organization. The table below highlights their key distinctions:
Why Implementing an AI Governance Framework Matters
The adoption of AI technologies introduces significant opportunities for innovation and operational efficiency. However, it also creates complex risks related to privacy, security, compliance, and ethical integrity. Without a structured governance framework, organizations often struggle to maintain visibility over how AI systems operate, what data they process, and how decisions are made. This lack of oversight can lead to regulatory fines, reputational damage, and unintended damaging outcomes.
An effective AI governance framework empowers organizations to establish clear accountability for AI initiatives, minimize legal exposure, and uphold public trust. It ensures that AI systems not only comply with regulations like the EU AI Act and ISO 42001 but also align with the organization’s risk tolerance and ethical standards. As AI adoption accelerates across industries, companies that proactively implement governance frameworks position themselves to innovate responsibly and sustain long-term success.
Key Principles of an Effective AI Governance Framework
Establishing effective AI governance requires a clear foundation built on core principles. These principles guide organizations in managing AI responsibly, ensuring outcomes are ethical, compliant, and aligned with long-term business objectives:
1. Clarity and Comprehensibility
Organizations must ensure that AI systems operate in ways that are easy to understand for both technical and non-technical stakeholders. This includes using plain language in governance policies and avoiding complex technical jargon when communicating AI decisions that impact employees, customers, or regulators. Clarity encourages trust and informed decision-making at all organizational levels.
2. Transparency and Openness
Transparent AI processes allow stakeholders to understand how models produce outcomes and what data sources are involved. Such transparency includes maintaining clear documentation of model development, decision logic, and evaluation criteria. Openness also involves sharing information about the limitations of AI systems and any steps taken to mitigate bias or performance issues.
3. Technical Resilience and Safety
AI systems must be designed to operate reliably under expected conditions and handle unexpected scenarios without producing harmful outcomes. This process involves regular performance testing, model validation, and risk assessments throughout the AI lifecycle. By prioritizing resilience and safety, organizations reduce the likelihood of unintended consequences that could affect business operations or customer trust.
4. Responsible Data Use and Privacy
The foundation of effective AI governance is responsible data management. Organizations should ensure that AI models are trained on high-quality, relevant data while adhering to applicable privacy regulations such as the GDPR and the EU AI Act. Data minimization, anonymization techniques, and clear consent practices help reduce regulatory risks and protect sensitive information.
5. Accountability and Role Ownership
Clear accountability ensures that every stage of the AI lifecycle, from model development to post-deployment monitoring, has assigned ownership. Defining responsibilities across legal, security, governance, and technical teams helps organizations quickly respond to issues and ensures ongoing oversight. Establishing dedicated roles or committees for AI governance reinforces a culture of responsibility and proactive risk management.
Established AI Governance Frameworks and Models
As AI adoption accelerates, several governance models have emerged to guide organizations in managing AI systems responsibly. These frameworks offer practical principles that help balance innovation with ethical and regulatory requirements:
The Hourglass Model
The Hourglass Model organizes AI governance into three key phases: data preparation, model development, and outcome management. Its structure emphasizes narrowing down data inputs to relevant, high-quality sources, applying rigorous controls during model creation, and continuously monitoring AI decisions post-deployment. This model ensures responsible AI use by maintaining strict oversight throughout the entire lifecycle of AI systems.
Google’s AI Governance Approach
Google follows a well-publicized set of AI Principles focused on fairness, accountability, and social benefit. These guidelines include prohibiting the development of AI for harmful or surveillance-related purposes and promoting explainability in AI outcomes. Google also emphasizes human-centered design, ensuring AI tools assist rather than replace critical human decision-making processes.
Singapore’s A.I. Governance (AIGA) Framework
Singapore’s AIGA Framework is one of the first national-level initiatives to formalize AI governance. It focuses on three pillars: human-centric AI, explainability, and accountability. The framework encourages businesses to adopt voluntary guidelines that promote trust in AI by ensuring responsible data use, transparent decision-making, and clear lines of accountability across AI development teams.
OECD AI Principles
The Organisation for Economic Co-operation and Development (OECD) developed five key AI Principles to guide responsible AI adoption globally. These include inclusive growth and sustainable development, human-centered values, transparency and explication, validity and security, and accountability. The OECD Principles are widely adopted across both private and public sectors to promote ethical AI use and reduce risks of harm.
NIST AI Risk Management Framework
Developed by the U.S. National Institute of Standards and Technology, the NIST AI Risk Management Framework provides structured guidance on identifying, evaluating, and mitigating AI-related risks. It emphasizes continuous risk assessments across the AI lifecycle and promotes documentation of AI system limitations, potential biases, and performance metrics. This framework is particularly useful for organizations managing AI in high-stakes environments such as healthcare, finance, and critical infrastructure.
What Regulations Require an AI Governance Framework?
As governments worldwide establish rules for artificial intelligence, organizations face growing pressure to meet regulatory expectations. While some frameworks are binding laws, others provide voluntary guidance. Understanding which applies to your business is critical for compliance planning:
Steps to Establish an Ethical AI Governance Framework
Building an ethical AI governance framework requires a structured approach that balances innovation with compliance and responsible practices. The following steps guide organizations through the process of setting up effective oversight for their AI systems:
1. Defining Objectives and Scope
Start by identifying what your organization wants to achieve with AI and the potential risks that need to be addressed. Clarify which AI technologies and use cases fall under governance oversight. This process ensures that policies are relevant, actionable, and aligned with business priorities.
2. Developing Governance Policies and Ethical Guidelines
Establish clear governance policies that outline acceptable AI use, model development standards, and responsible data practices. This task requires developing strong AI data governance protocols to manage data lineage, user rights, and the handling of sensitive information throughout the AI lifecycle. Include ethical guidelines that address fairness, accountability, and transparency to guide both technical teams and business leaders.
3. Assigning Roles and Responsibilities
Clearly define who is responsible for managing AI governance activities. This includes assigning ownership for policy enforcement, compliance monitoring, and addressing incidents related to AI systems. A well-defined ownership model reduces confusion and accountability gaps.
4. Implementing Training and Change Management
Ensure that employees understand the organization’s AI governance policies and ethical standards. Provide training programs tailored to different roles, from AI developers to senior leadership. Promote a culture of responsible AI use by embedding governance awareness across the organization.
5. Setting up Monitoring, Audits and Review Cycles
Establish continuous monitoring to assess AI system performance, compliance status, and potential risks. Schedule regular audits and review cycles to keep governance policies aligned with evolving regulations and business objectives. Use findings from these reviews to refine governance practices over time.
Tools & Technology Solutions for AI Governance
A modern AI governance framework is impossible to manage without specialized tools that automate oversight and ensure compliance. These solutions support everything from risk assessments and policy enforcement to model transparency and ethical evaluations.
Policy Automation Platforms for AI
Policy automation platforms help organizations implement governance controls at scale, transforming internal policies into actionable enforcement mechanisms. MineOS offers a no-code system for automating policies that enables legal, privacy, and compliance teams to configure AI usage rules and apply them consistently across business units.
Risk & Impact Assessment Tools
Risk and impact assessment tools evaluate AI models for ethical, legal, and operational risks before deployment. MineOS simplifies this process with automated assessments that map regulatory obligations, including the EU AI Act and ISO 42001, to specific AI use cases, reducing manual workload and improving accuracy.
Data Lineage and Traceability Solutions
Understanding how data flows through AI systems is essential for accountability and risk mitigation. Data lineage tools visualize these flows, helping organizations identify where personal or sensitive data is used, modified, or stored throughout the AI lifecycle.
AI Model Inventory and Registry Systems
Maintaining a centralized inventory of all AI models and datasets is a key governance requirement. Registry systems ensure organizations have full visibility into sanctioned and unsanctioned (shadow) AI assets, enabling proactive compliance management.
Consent and Preference Management Tools
These tools capture, store, and manage user consent for data usage in AI models. Effective consent management ensures that AI systems respect individual privacy preferences and remain compliant with data protection regulations.
Monitoring and Drift Detection Technologies
AI models can experience performance degradation over time, leading to inaccurate or biased outcomes. Monitoring tools equipped with drift detection capabilities identify when models deviate from expected behavior, allowing teams to intervene before harm occurs.
Model Explainability and Auditability Tools
Explainability solutions help organizations demystify complex AI models by providing clear, understandable insights into how decisions are made. These tools are essential for high-stakes environments where explainability is a regulatory or ethical requirement.
Compliance Mapping and Regulatory Alignment Software
Compliance mapping tools translate complex regulatory requirements into actionable governance activities. MineOS excels in this area by aligning AI operations with international standards such as the EU AI Act, NIST AI RMF, and ISO 42001. Its built-in templates and audit-ready reports simplify compliance and reduce regulatory risk.
Role-Based Access and Accountability Tracking
Maintaining proper access controls and accountability is critical for reducing AI misuse and unauthorized model changes. Clear ownership and well-defined permissions help organizations stay compliant and minimize operational risks.
- Identifying and Assessing AI-Specific Risks: MineOS provides dedicated capabilities for building and maintaining an AI risk registry, helping organizations identify, document, and monitor emerging AI-related risks across the enterprise.
- Mitigating Bias, Drift, and Unexpected Outcomes: Implement controls to detect and correct model drift, data quality issues, and unintended bias before they impact critical decisions.
- Ensuring Systemic Security and Data Privacy: Apply strict access controls and security policies to protect sensitive datasets and AI models from unauthorized access.
- Managing Model Explainability and Accountability: Ensure AI decisions remain understandable and traceable by assigning clear ownership to AI models and their outputs.
Best Practices for Maintaining an AI Governance Framework
Sustaining an AI governance program requires more than initial implementation. Organizations must adopt practical strategies that keep governance frameworks effective, relevant, and aligned with evolving technologies and regulations.
- Securing Executive and Leadership Buy-In: Ensure long-term success by aligning AI governance initiatives with strategic business objectives. Engage executives early, demonstrate measurable value, and incorporate governance goals into broader corporate governance agendas.
- Continuous Team Education and Training: Build organization-wide awareness of AI risks, compliance obligations, and ethical standards. Offer regular training sessions to privacy, legal, security, and technical teams to keep them informed about new regulations and governance practices.
- Real-time Monitoring and Incident Response: Establish continuous oversight of AI models to detect issues such as model drift, bias, and compliance failures. Develop clear incident response procedures that assign ownership and define immediate corrective actions when governance breaches occur.
- Documentation, Auditing, and Version Control: Maintain detailed records of governance activities, including policy changes, risk assessments, and incident reports. Implement version control to track governance framework updates and ensure audit readiness at all times.
- Iterative Updates in Response to Tech and Policy Change: Review and refine your AI governance framework regularly to stay ahead of emerging technologies and evolving regulatory landscapes. Incorporate lessons learned from audits and real-world incidents to continuously improve governance effectiveness.
Pros and Cons of Implementing AI Governance Frameworks
Adopting an AI governance framework brings clear advantages but also presents operational challenges. Planning and managing expectations are made easier for organizations when both sides are understood.
How MineOS Enables AI Governance Frameworks
MineOS provides a comprehensive platform designed to help organizations implement and maintain effective AI governance frameworks. By automating compliance processes, offering real-time visibility into AI assets, and aligning operations with global regulations, MineOS simplifies the complexities of AI governance.
- Automated AI Asset Discovery: MineOS automatically identifies AI tools, datasets, and platforms within an organization, including generative AI tools like ChatGPT and internal AI projects. This procedure ensures full visibility into the AI ecosystem and aids in compliance efforts.
- Risk Assessment and Compliance Alignment: The platform offers customizable assessment templates to evaluate AI vendors and projects, aligning with frameworks such as the EU AI Act, NIST AI RMF, and ISO 42001. Continuous monitoring helps organizations stay ahead of compliance risks.
- Policy Enforcement and Access Controls: MineOS enables organizations to set and enforce data policies, including data retention and privacy-by-design standards. Role-based access controls ensure that only authorized personnel can access sensitive AI models and datasets, supporting accountability and reducing risks.
- Continuous Data Mapping and Classification: With AI-powered classification, MineOS provides real-time, accurate views of sensitive data, automating tagging and categorization to eliminate repetitive privacy tasks. This approach supports compliance with regulations like GDPR, CCPA, and the AI Act.
By integrating these capabilities, MineOS empowers organizations to adopt AI technologies responsibly, ensuring compliance and promoting trust in AI-driven initiatives.
Conclusion
AI has moved beyond experimental projects and now plays a central role in shaping business strategies and societal outcomes. With this growing influence comes an urgent need for structured, ethical, and transparent governance. Organizations that establish clear frameworks for managing AI will be better positioned to mitigate risks, build long-term trust, and unlock the full potential of these transformative technologies.
While implementing an AI governance framework requires thoughtful planning and continuous oversight, the cost of inaction is far higher. Regulatory landscapes are evolving rapidly, and public expectations around responsible AI use continue to rise. By taking a proactive approach today, organizations can lead with integrity, navigate future challenges with confidence, and turn responsible AI adoption into a lasting competitive advantage.