A Practical Guide for AI & Data Privacy Compliance

%20(1).png)
Getting Practical About AI Privacy
The emergence of the General Data Protection Regulation (GDPR) in Europe and the California Consumer Privacy Act (CCPA) in the United States marked a significant shift towards recognizing and safeguarding the rights of individuals over their personal data, but only 6 years after the launch of GDPR, AI's rise has pushed the envelope on what privacy means.
AI's knack for data analysis and decision-making upsets the limits of data minimization and consent, introducing gray areas in compliance and governance. For this reason, regulations such as the EU AI Act are here and more are imminent, making it imperative that enterprises deploying or using AI tools understand their responsibilities. Together, we’ll explore the requirements of AI regulations and data privacy legislation and discuss how to manage privacy and governance in this new world.
AI Extends the Privacy Role
Data protection has evolved significantly, with AI- related risks and responsibilities seen as a recent capstone to what began not too long ago as data security and then data privacy. While external threats were (and still are) a concern, as technology and use cases changed, we started to look inward at the appropriate internal usage of data. That idea of “privacy engineering” is relevant today, but with new at-scale use cases for data (not the least of which is AI tools), at-scale decision making is now a necessity, and so the idea of AI governance and its new rules, regulations and frameworks are born.
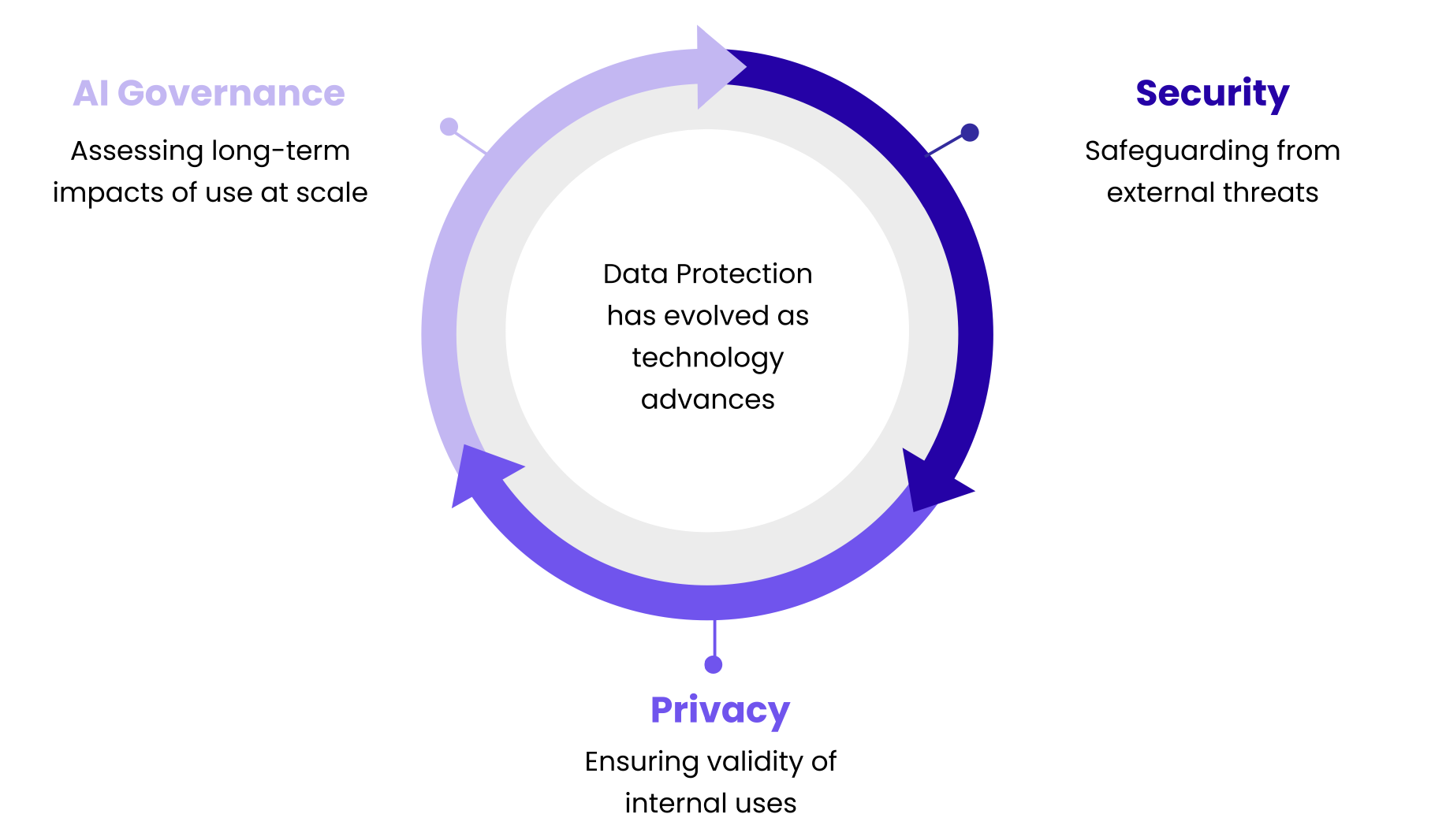
Scale Decision Making to Meet Your AI Requirements
The good news for privacy, security and IT professionals is that these new ideas are merely an extension of what was previously built on, not a rewrite of the entire privacy playbook. Creating an environment with ethical and compliant AI data usage does not require many new responsibilities in the enterprise, though the degree to which the job changes does depend on whether your company is an AI user or an AI developer (or both).
What is the EU AI Act?
The European Union's AI Act marks a strategic regulatory approach to the evolving AI landscape, reflecting a deep engagement with both the potential and perils of advanced technologies like ChatGPT. This legislative framework shows the EU's ambition to foster innovation in the AI and data privacy space while offering a blueprint for responsible AI development.
A Balanced Approach
The Act exemplifies how the EU recognizes that for AI to succeed as a concept it must straddle the line between promoting innovation and ensuring the protection of individual and environmental rights. It takes a multifaceted risk-based approach to do so.
Risk-Based Classification
The Act classifies AI into categories that define the level of risk of various AI applications and change the level of enforcement and oversight required by companies depending on the types of AI they use or deploy.
Unacceptable Risk: Bans AI systems that threaten democratic values or personal autonomy, with exceptions for critical uses under strict conditions>
Example: AI systems that compromise people's safety or rights, including social scoring, exploitative AI targeting vulnerable groups, and indiscriminate surveillance, are banned due to their severe threat to individual freedom and safety.
High Risk: Demands thorough vetting for AI in critical infrastructure or consumer safety, emphasizing public welfare and human rights
Example: These AI systems, crucial in sectors like critical infrastructure, employment, law enforcement, and biometric identification, require strict compliance before deployment due to their potential impact on public safety and fundamental rights.
Limited Risk: Calls for transparency in AI interactions that might impact user experience, prescribing lesser regulatory oversight
Example: AI applications such as chatbots and AI-generated content, which necessitate transparency to ensure users are aware they're interacting with AI, aiming to prevent deception and misinformation.
Generative AI/Systemic Risk: Not explicitly categorized but subject to relevant obligations based on application, focusing on transparency and misuse prevention}
Example: Most AI applications, including spam filters and AI- enabled video games, pose little to no risk, allowing them to operate freely to encourage innovation without significant regulatory constraints.
What Are the Implications for Businesses?
The most pressing issue for businesses is when and how they need to get compliant with the Act. Enforcement is expected within a 12–24 month timeline, urging businesses to swiftly align their AI strategies with regulatory requirements.
In essence, businesses are called to adapt quickly, and consider what the requirements for governance are specific to their AI operations; the type of governance required falls on a scale from the lowest risk (AI-enabled SaaS) to the highest (internal AI development for high impact use cases), and at present, most enterprises whose AI use stops at generative AI usage (like ChatGPT, which lies right in the middle of the risk spectrum) will find that their compliance efforts won’t need to extend as far as AI developers, necessitating little beyond the types of privacy operations they’re currently undertaking for GDPR, CCPA, etc.
But it’s important to first grasp AI usage in the enterprise and then determine next steps from there.

An AI Workflow Can Cover Your Bases
There are some common, solid frameworks and guidelines that cover the specific AI data privacy requirements. The workflow below is loosely based on ISO 42001, and provides a reference for meeting organizational objectives and addressing risks related to the design and operation of AI systems. Not all the control objectives and controls listed are required, and generally a company can design and implement their own controls as long as the general bases are covered.
1. AI Accountability and Org Policy
This framework distills the essence of effective AI management into three actionable areas, focusing on policy alignment, organizational accountability, and lifecycle vigilance, without tethering to specific standards.
Policy Alignment for AI Management
Companies must craft dynamic policies that guide AI system management in harmony with overarching business goals. Such policies should serve as a blueprint for AI initiatives, ensuring they complement and amplify business strategies. The goal is to create a flexible yet robust policy environment that propels AI systems towards delivering tangible business outcomes
- Relevance Across Risk Levels: This area is universally applicable, vital for managing AI systems at any level of risk.
Organizational Accountability in AI
This involves clearly defining roles, responsibilities, and pathways for decision-making, especially in scenarios where AI's implications are profound. Cultivating an environment of transparency and responsibility ensures that AI technologies are leveraged ethically.
- Critical for High and Unacceptable Risk: The need for well-defined organizational accountability becomes larger as the potential risk associated with AI systems escalates.
Lifecycle Management of AI Systems
This guideline entails establishing processes that oversee the ethical design, deployment, and eventual sunsetting of AI systems. By prioritizing lifecycle management, companies can safeguard against ethical pitfalls and align AI practices with both internal values and external expectations
- Essential Across All Risk Levels: While important for any AI system, lifecycle management is particularly crucial for those posing high and unacceptable risks, meaning in-house development and training of AI models.
By emphasizing policy alignment and lifecycle management, companies can mitigate risks and demonstrate that the organization is accountable where necessary.
2. ID Your AI Stakeholders
When ensuring compliance with AI regulations, a critical step is identifying all stakeholders involved in the AI lifecycle. This includes recognizing and recording the roles and expertise of those responsible for using, creating, deploying, operating, managing changes, maintaining, transitioning, and retiring the AI system, in addition to tasks related to its verification and integration.
Essentially, it's about mapping out who does what across the entire span of the AI system's existence, and highlighting the roles and responsibilities that contribute to its lifecycle. This is relevant even for companies using vendor-provided AI systems, and something that a comprehensive data map solution will be suitable for, as it can help gain visibility over all types of AI tools and even AI models or datasets (at the higher end of risk), as well as those using and deploying them.
3. AG AI Impact Assessments
- Objective 1: In-Depth Evaluation of AI System Effects
The first goal of conducting AI assessments is to evaluate how AI systems influence individuals, groups, and broader societal dynamics. Though this objective is more critical when dealing with AI applications classified under High and Unacceptable Risk categories, some form of impact assessments are important at every risk level of AI usage.
You’ll want to have an inventory of AI assets and understand in-depth the way that these assets process data and why. A great starting place is a data map which details who has access to AI systems, what these systems do with data, and make it simple to record why they’re within the scope of justified processing.
Understanding the risks associated with the AI tool and what the potential impacts of these risks might be will be critical at this stage, and will also sound familiar if your company already does DPIA reports, which for most lower risk AI systems work suitably to cover this requirement, and manage to mitigate risks effectively.
Risk Relevancy: Targets High and Unacceptable Risk categories, though detailed impact assessments to understand and mitigate potential adverse effects, and processing justification should be recorded for all AI assets in every risk level.
- Objective 2: Comprehensive Resource Accounting for AI Systems
The second objective emphasizes the importance of meticulously accounting for the resources that are crucial to the operation and sustainability of AI systems. This includes a broad spectrum of inputs, from computational resources to human expertise, necessary for the development, deployment, and maintenance of AI technologies.
Data maps are key to the transparency of AI data usage, and critically, who is using them, for what purpose and how. A good data map can even record which datasets an AI model has access to, where an AI being trained can draw data from, and what data types are the output.
4. Discover Data within AI Systems
We can split the requirement to discover the data within AI systems into two parts. While the basics of data mapping can capably cover the previous requirements for justified AI data processing, stakeholder and asset identification and more, you’ll want an in-depth solution to understand which data types the AI is processing.
- Objective 1: Visibility and Management of Data in AI Systems
The goal of this objective is to achieve an understanding of the role data plays in AI systems and to maintain diligent management of this data throughout the systems' lifecycles. This requirement is crucial across all levels of AI application risk, yet it becomes particularly paramount when dealing with High-Risk categories. The emphasis on High Risk arises from the challenges of handling sensitive data and its substantial influence on the outcomes and integrity of AI systems.
Data discovery is critical for several use cases including data privacy compliance, security and more. You want to scan for sources of data processing in as many ways as possible, with your goal being 100% coverage of all data sources in use by the company, a way to delineate which systems are categorized as AI, and a way to exhaustively yet economically understand what types of data are where. This can be time consuming, especially for internal, custom tools so using a sophisticated data discovery method is recommended.
- Objective 2: Third-Party and Customer AI Interactions
The second objective focuses on the management of data responsibilities in dealings with third parties and customers concerning AI systems. It is relevant to all risk categories spelled out in the EU AI Act, with an enhanced focus on High and Unacceptable Risk levels, to guarantee that external entities engage with the organization's AI systems under the umbrella of responsible AI practices.
Third-party and customer interactions should be governed by clear guidelines and expectations, promoting transparency and accountability. Assessment of third party risk and the role of third parties and customers in any data environment involving AI helps prevent misalignments and ensure that all parties involved are contributing positively toward responsible and compliant AI usage.
Use Cases Frame Next Steps
If your AI use cases have already been assessed for privacy impacts and inclusion in a DPIA - which is most relevant to companies whose AI use doesn’t extend beyond generative AI - the EU AI Act requirements may be more familiar than you think. For developers of AI tools and those training AI models, a bit more scrutiny of your practices is required.
The Conformity Assessment
A conformity assessment is not expansively different from the nature of DPIA and similar data assessments, though the assessment and declaration of conformity will be required of those companies that are developing High Risk AI models. These assessments are crucial for demonstrating that AI systems meet legislative criteria before they are introduced to the market or used within the EU. A new conformity assessment must be conducted if significant modifications are made to a High Risk AI that could affect its compliance or alter its intended purpose.
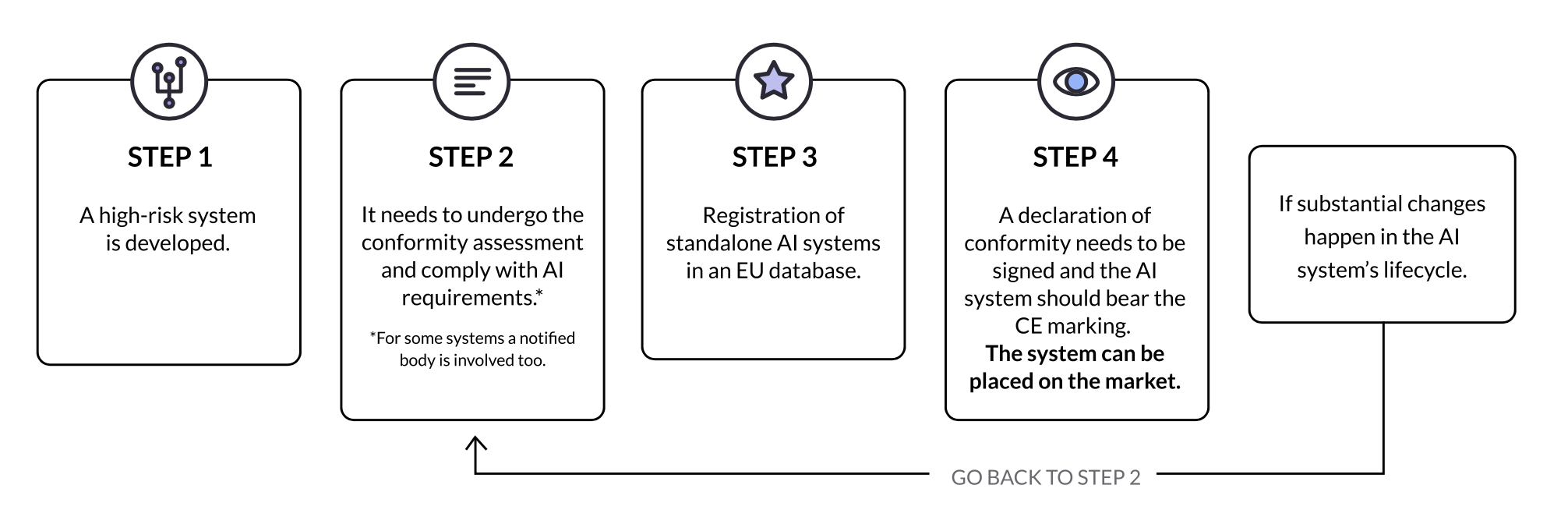
Thankfully, it’s a simple matter to take questions from the conformity assessment and append them to existing documentation and processes:
Start to put yourself in-scope for some of the EU AI Act requirements and other impending legislation by taking a good look at your legal contracts. Many vendors and other third parties are sending AI addendums to their standard agreements specifically about leveraging data to improve the product or service (with AI in mind). You may want to consider attaching limitations to those addendums, for example to specify that usage of data is disallowed for those High Risk AI use cases.
You might also want to alter the privacy reviews that occur during procurement and during new product design and development, programming in some of the things that need to be addressed in the conformity assessment in order to save yourself time and cover your bases.
Externally, it’s also a good idea to update user- and customer- facing collateral with content addressing AI and data usage. Ensure that your code of conduct discusses the ethical usage of AI as well.
Action Items
Regardless of whether your company uses a few AI-enabled SaaS platforms and a few employees have access to ChatGPT, or whether your organization develops new AI models, the idea of protecting the data lifecycle remains the same. AI does not do anything different with data than what was being done prior toAI, it is only doing it at a much larger scale and much more autonomously. The same decisions about what is and what is not allowed still need to happen, but these decisions now need to happen with the scale and agility matching AI. That is the difficult part.
It’s critical to get visibility and be able to audit the entire data lifecycle, even as it expands to larger proportions due to the inclusion of AI. Around the auditing capability must go the ability to control what is happening, enforce ethical data usage by AI tools and models with policies that are both recorded on paper and put into action.
The first step is to start cataloging AI related assets.While companies that are categorized as users of AI tools will need to do a census of stakeholders and AI-enabled SaaS tools, the assets get more varied for companies that deploy or develop AI systems.
MineOS AI Governance Tools
MineOS specializes in AI Asset discovery, cataloging, assessments and governance. Our industry-best data mapping solution addresses the AI compliance requirements of organizations at any level of AI risk.
We help you to justify these AI tools’ usage of data in accordance with new and emerging regulatory frameworks, and address these frameworks’ requirements to determine and estimate AI risks and to establish AI controls.
Fast visibility, control and compliance for all the AI tools you use; MineOS AI Asset discovery scans your SSO tool, corporate email inbox and cloud infrastructure to uncover:
- AI developer tools: And other indications you’re developing AI
- Generative AI Vendors: Like OpenAI, used for productivity and development
- AI-enabled SaaS: Tools that leverage AI for certain features. We automatically assign their purpose, risks & assessment
- Internal AI/ML Projects & AI Datasets: We can easily integrate into and then scan these file
- ... and who on your team is using them.
A Single Source of Data Truth with granularity that fits your AI use cases
MineOS automatically recognizes the purpose and regulatory frameworks relevant to your AI tools, and provides risk and other assessment templates to help you justify your use of AI tools.
Use our AI assessment builder to create custom assessments for your internal AI/ML projects and initiatives.
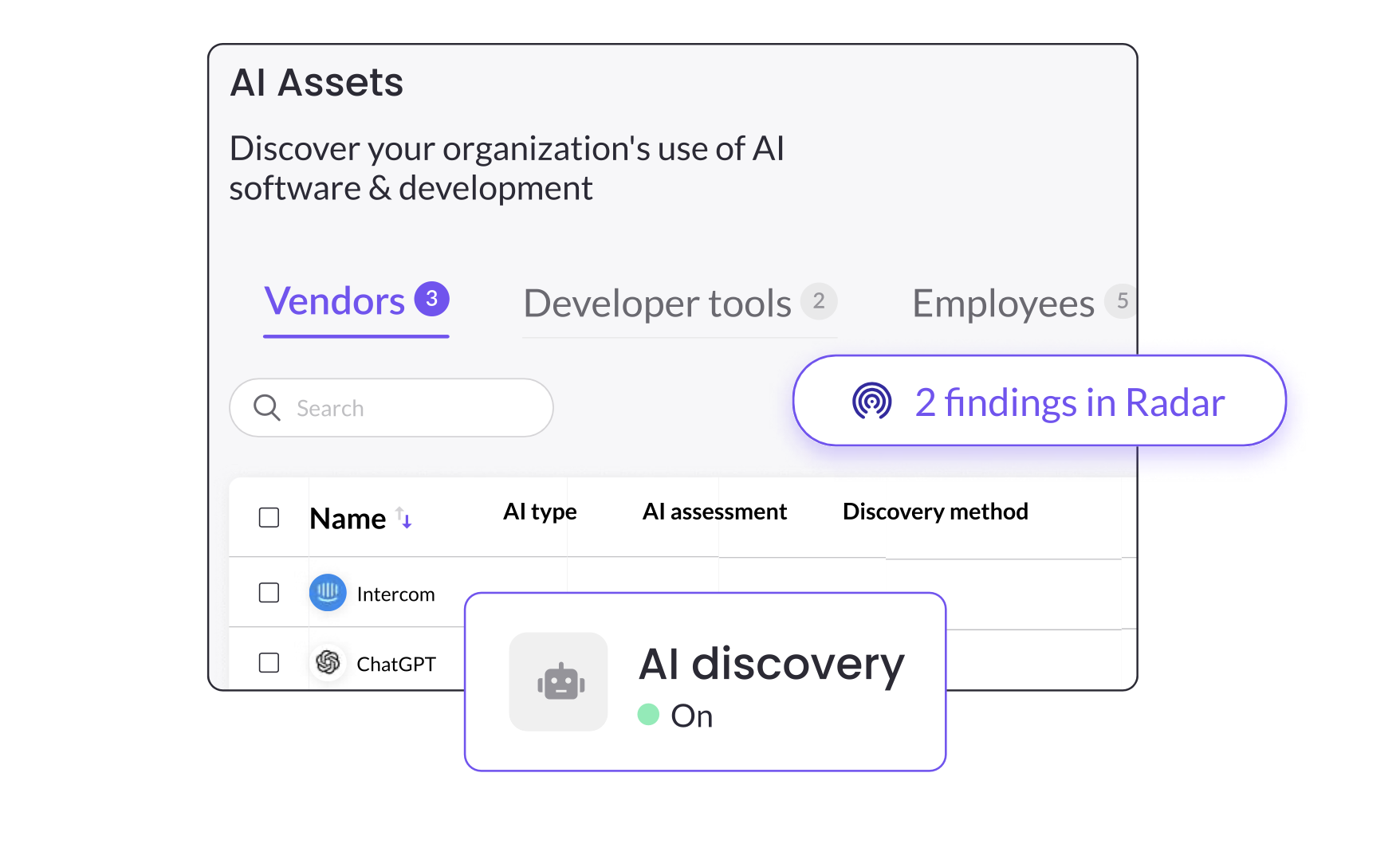
the lowest risk (AI-enabled SaaS) to the highest (internal AI development for high impact use cases), and at present, most enterprises whose AI use stops at generative AI usage (like ChatGPT, which lies right in the middle of the risk spectrum) will find that their compliance efforts won’t need to extend as far as AI developers, necessitating little beyond the types of privacy operations they’re currently undertaking for GDPR, CCPA, etc.
But it’s important to first grasp AI usage in the enterprise and then determine next steps from there.
Vendor risk reports help visualize risks associated with 3rd-party tools and AI. Recognizing your unique risk factors and being able to assess and mitigate them is a critical step towards compliance.
Govern your AI privacy program with policy rules that keep AI from using forbidden data, and notify you if noncompliant data types are found. By accomplishing that, you'll have a baseline that stands up to any AI regulation.