Assessing the Risk of AI Assets
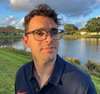
.png)
Shadow IT can be the bane of any organization. Unauthorized and unreported data systems bloat data stacks, waste resources, and open up companies to needless risk. All of this translates to a lack of control, which is why visibility is so vital to business today.
AI-powered tools like ChatGPT, Gemini, and a wave of what’s soon to come will only exacerbate this problem and highlight the need for unprecedented oversight of an organization’s data stack.
The Risk of Shadow IT
Any data source carries risk, with the level of risk typically depending on if a data source has access to personal or sensitive information and how much of that data lies within the system.
Those problems are amplified when systems are used without approval from the organization or worse, the person who signed up for the software forgets about it but never cancels it. This is a common case in any company, as anyone who has put together a data map manually can attest to.
While tools to automate data mapping have brought major developments to the privacy sphere in the past several years, many still conduct data maps manually by interviewing departments and staff to see which systems they use and why. That approach leaves a massive hole in data discovery, as MineOS’s stats back up. Our average customer discovers at least 30% more systems than they initially report when conducting a data map with our technology.
Considering one of the most commonly exploited vulnerabilities in data breaches is outdated software, having Shadow IT full of outdated versions of systems creates a massive vulnerability.
Beyond entry points to data breaches, Shadow IT provides quieter opportunities for harm as well, as systems that IT has no knowledge or control of could be used to siphon off customer data, a major compliance problem.
Shadow IT and AI Risks
These vulnerabilities are real given the vast scope of most organization’s data stacks today, as the average organization uses hundreds of SaaS apps. But AI, even in small doses, complicates this picture exponentially.
The most obvious AI risk is new weapons bad actors and cyber criminals will have access to, a point which keeps CISOs up at night. In a recent CISO report, 70% of CISOs said they feared generative AI would give cyberattackers the upper hand.
IT may be well prepared to try and counter these attacks, but the average non-IT employee will likely not be, stretching the difference between secure and unsecure points within an organization to a gap wider than ever before.
But despite the flashiness of cyber attacks, that is far from the most severe AI risk. In reality, AI risks will live within organizations themselves–through unauthorized staff AI use and unintentional mistakes made when using AI.
This all traces back to the introduction of ChatGPT, but as more AI systems become widely used, the problem will spread.
Large language models like ChatGPT and other text-based AI systems are collecting and training on immeasurable amounts of data, including virtually everything consumers put into it. The developers behind these systems have had trouble explaining precisely how they process user data, which is part of the reason there has been so much scrutiny from data privacy regulators, including Italy’s regulator has contemplated a ban on ChatGPT.
This first wave of advanced AI systems was not created with guiding principles such as privacy by design or data minimization. ChatGPT’s impenetrable DSR process and a multitude of AI chatbots that collect data without notice and offer weak user privacy protections have proven that over and over.
If AI does not provide the safety nets needed to value privacy and data, then using them is a risk in and of itself. Despite pleas from companies not to ever input any sensitive information into ChatGPT or Gemini, many workers are ignoring those requests to try and streamline their duties, creating substantial and unseen risk.
Training can only do so much when the presence of AI itself exacerbates risk, and when organizations are not able to detect and monitor which AI systems are being used and how they are being used, they are powerless to stop bad behavior, even when done unintentionally. That fact is what broadens Shadow IT when dealing with AI systems, and privacy and security professionals need to be able to address that in compliance and confidence.
The Solution to AI Risks
The primary way to mitigate AI risks then is to ensure proper oversight over your data stack, making data mapping a backbone of not just privacy, but cybersecurity in 2024.
By eliminating as much Shadow IT as possible, an organization can minimize the traditional risks associated with it while also honing in on AI-powered data systems. Using an advanced data mapping tool like MineOS can not only discover and classify data with near complete accuracy, but it can uniquely identify AI systems in use so an organization can create governance layers on top of them to manage those inherent risks and misuses.
That way, you can ensure employees are not using prohibited AI systems, and for the AI systems that are in use, you can provide additional guidance–with the proper oversight–to combat the built-in privacy deficiencies of so many AI programs.
The AI revolution is here, and we all need to be mindful of privacy as we begin to embrace it. Visibility is the first step towards control, so see how MineOS’s AI data discovery works for yourself.